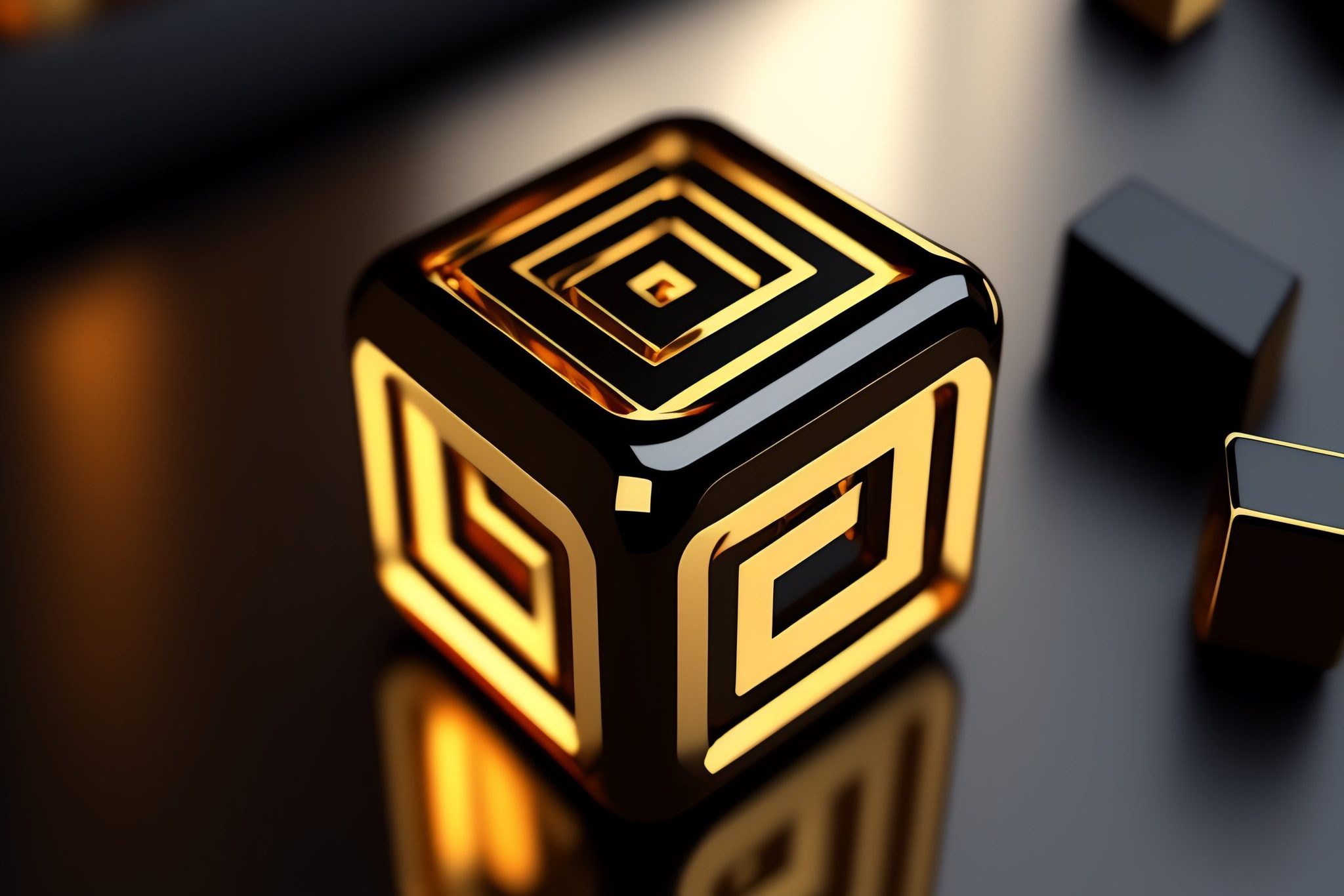
Introduction
Foundation models are a new class of powerful AI systems which are having an unparalleled impact on both machine learning research and industrial applications. I recently attended the Exploring foundation models symposium hosted by The Alan Turing Institute. This event brought together prominent academics and practitioners to explore the promises, limitations and future directions of this rapidly developing technology.
Insights into foundation models
Welcome and introduction
Professor Michael Wooldridge kick-started the event with an overview of foundation models. He focused on contemporary large language models such as GPT-3 and ChatGPT. Michael also briefly shared the key characteristics which make these models stand out. Some of these are parameter size and heavy training requirements. To conclude, he covered their current limitations e.g hallucinations and propensity for bias.
Highlight
- The Alan Turing Institute’s aspiration is to lead the UK in the development of a sovereign AI resource
The evolution of large language models
Professor Phil Blunsom explained the basics of how language models work (language models assign a probability to a sequence of words) before moving on to the mechanics of the transformer architecture. Transformers were developed in 2017 and are responsible for the recent innovations in natural language processing. Phil then noted the challenges of model evaluation given that LLMs are trained on almost all standard datasets.
Highlight
- Base model training is data inefficient (approx 1 trillion tokens) and expensive (0.5 exaflops of compute over 1-2 months)
- Supervised training of LLMs via instruction following is more data efficient but is expensive due to the expense of human annotation
Related work
- A Systematic Investigation of Commonsense Knowledge in Large Language Models
- Learning and Evaluating General Linguistic Intelligence
Challenges faced by PLMs and mitigation strategies from knowledge enhancement and evaluation
Professor Maria Liakata started by examining the strengths of pre-trained language models (PLMs) such as BERT and GPT-3. PLM strengths include their ability to capture higher-order co-occurrences in text and their creative writing capability. Maria also revealed challenges including privacy concerns (most PLMs are close-sourced) and LLMs’ poor ability with long sequences. The trouble with long sequences is particularly clear when it’s necessary to capture important events and preserve temporal order in generated summaries.
Highlight
- PLMs knowledge is enhanced via implicit and/or explicit incorporation strategies
- Some implicit strategies are knowledge-guided masking & knowledge-related pretraining
- Explicit strategies include model input modification, knowledge fusion and knowledge retrieval
Related work
Human-centric benchmarking of foundation models for trustworthy deployment
Katie Collins’ presentation showcased the design of a new benchmark for foundation models. LLMs have a tendency to learn predictable responses from the language they’ve seen before. Katie’s work constrains the language LLMs can produce when answering goal-based planning and casual explanation prompts. This is then compared to human performance on the same tasks resulting in an effective benchmark for measuring the reasoning capability of LLMs.
Highlight
- Humans outperform LLMs across both planning and explanation tasks with constraints
Related work
Hierarchical3D adapters for long video-to-text summarisation
Professor Mirella Lapata walked us through the intricacies of the summarisation task. Some of the key challenges included long input sequences (115-minute videos with roughly 23,000 words), complex semantics, evaluation (what makes a good summary) and data scarcity. She explored how these considerations could be addressed with novel datasets such as SummScreen3D and parameter-efficient models to handle data scarcity.
Highlight
- It’s possible to augment summarisation with architectures that combine multiple modalities
Related work
Some challenges for foundation models
Professor Anthony Cohn’s talk was a whirlwind tour through the failure modes of foundation models on common sense reasoning tasks. His experiments showcased how ChatGPT faired miserably across various types of Winograd schemas to reasoning about latitudes, cooking and liquids! These examples showed how little real-world understanding can be derived purely from training on text data.
Highlight
- We must still be cautious when using any outputs from foundation models and thoroughly benchmark their performance
Related work
Foundation models that can tell us when they don’t know
Professor Yarin Gal then introduced research which makes foundation models more useful. In this work, LLMs are imbued with the capability to request more information when presented with questions they can’t confidently answer. Humans naturally ask clarifying questions when presented with vague requests. Yarin demonstrated the CLAM framework for question-answering tasks which resulted in an increase from 34.25 to 54.4 in the model’s adjusted accuracy.
Highlight
- Introducing forms of metacognition (thinking-about-thinking) into LLM deployments provides a pathway to greater trust in these systems
Related work
Holistic evaluation of language models
Professor Percy Liang concluded the series of talks with a presentation on HELM which is a comprehensive benchmark suite for foundation models across multiple dimensions. The initiative aims to bring greater transparency to large language models and simultaneously inspire more responsible model development. This is achieved via systematic exploration of scenario tasks, multi-metric measurement (which includes accuracy, robustness, collaboration and more) and standardisation across both closed and open-source models.
Top highlight
- HELM is a continually improved tool with transparent results which can accelerate model comparison and selection for LLM-based research and products
Related work
Building on the foundations: where does AI go from here?
The symposium culminated in a panel with all the above speakers plus Dr Laura Gilbert. Questions ranged from the future research directions of foundation models to breakthrough commercial applications. One recurrent theme was the barrier presented by closed-source models. These models make it difficult to undertake thorough research because details regarding the training data and methodologies are unknown. Additionally, many of these models are only exposed via an API. Furthermore, training similar models is currently infeasible for academic institutions due to the astronomical cost of compute. These characteristics are a significant bottleneck to foundation model research. All the panellists were unanimous in their support for a future where foundation model capabilities are more open and accessible to humanity.
Wonderful conversations
The content of the Exploring foundation models symposium was phenomenal. However, the conversations I had during various breaks made the event truly memorable for me:
- It was fascinating to hear from Euan on how banks are integrating multimodal data to better serve their customers. We also spoke about the importance of tenacity, rapid learning and focus for success in an increasingly technological world
- Professor Nigel and I turned out to be sceptics of AI consciousness. It doesn’t seem obvious how AI systems will obtain consciousness to the same extent as human beings (after all, intelligence is not the same as consciousness). Nigel has recently published Rise of the moral machine: Exploring virtue through a robot’s eyes which I can’t wait to read
- With foundation model integration becoming more prevalent in real-world systems, I was delighted to discover that James and Gianluca are building BountyfulAI. This will enable practitioners to interrogate foundation models across various metrics. Latency and cost are crucial factors in production systems and their tech will facilitate smooth switching between providers
- Dr Shyam graciously informed me of the work he’s doing at The Turing Institute to imbue AI systems with an ethical framework. We touched on the impossibility of creating one single satisfactory framework for all potential users of a system. Given the variability in human preferences, it’s paramount that AI systems are customisable to allow for differing value systems
- I enjoyed speaking with Bethany from Faculty about the limitations of foundation models. It was also great to chat with Pradnya and Jay about the data science market and their experience of doing a master’s degree in Applied Artificial intelligence
Conclusion
I had a marvellous time at the foundation models symposium. I would especially like to thank The Alan Turing Institute for granting me a place to attend via their Access Fund. Special mention goes to Professor Michael Wooldridge, Ruth Drysdale and Katy Thompson who organised the event. I appreciate all the speakers for sharing their research. I now have a comprehensive view of the possibilities and limitations of foundation models. My knowledge base is more grounded in reality instead of hype.
However, It’s evident that we’ve reached a turning point in machine learning system capability. Following the symposium, I’m even more invigorated to ensure these models serve the highest good of humanity on both micro and macro scales. The future of AI is truly bright!